Keeping track of Internet latency is a classic measurement problem. Open measurement platforms, such as RIPE Atlas, offer geographically distributed, publicly accessible devices from which anyone can run active measurement campaigns and even leave behind open data. But, they also face significant challenges; for example, they require the distribution and maintenance of measurement devices all over the Internet.
We see an opportunity to complement open measurement platforms with an unconventional data source: gaming footage. Gaming is very latency-sensitive – the fact that searching for “how to lower ping/lag” yields hundreds of thousands of results is indication enough. In light of this, many video games display the latency between the player and the game server on-screen (Figure 1).
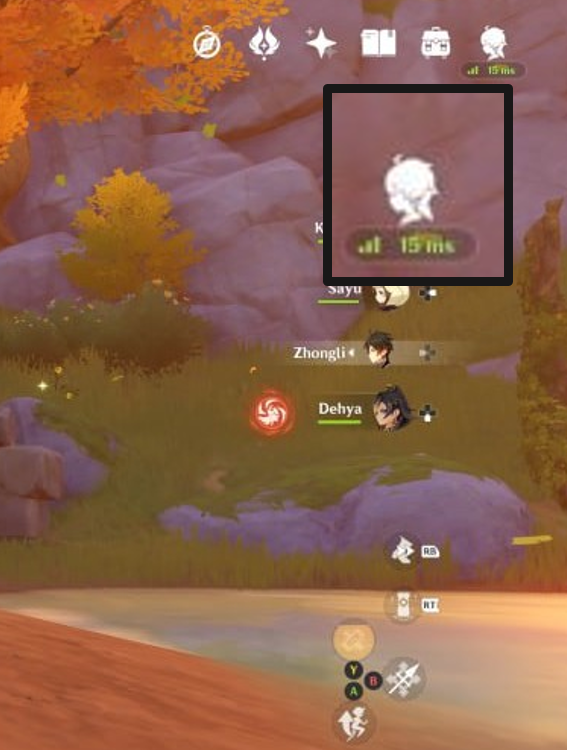
This in-game latency display offers a unique combination of features: It captures the latency experienced by users daily and can be obtained without intrusive traffic capturing and without introducing extra (measurement) traffic in the network.
With these observations in mind, my colleagues and I at EPFL, Lausanne, looked for publicly available video game footage to extract latency information. That was easy to find: streaming gaming sessions has become a popular hobby and profession.
How do we Extract Latency from Gaming Footage?
We developed Tero, a system that extracts latency numbers from publicly available images made available by the Twitch streaming platform on their official CDN. After more than two years of operation, Tero has processed nearly 400 million gaming images, obtaining almost 100 million latency measurements from 184,000 streamers globally (Figure 2).
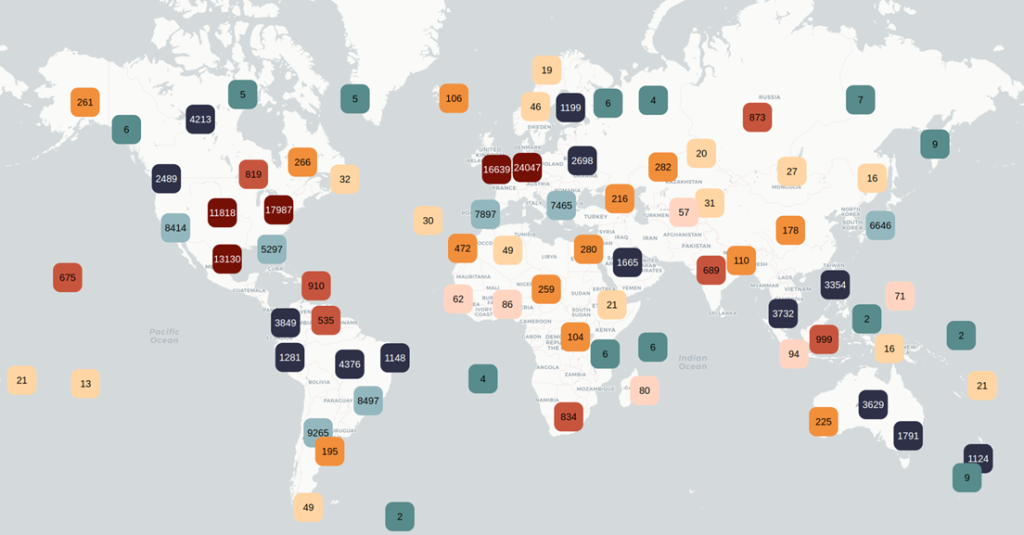
Tero performs two main functions:
- Extracting latency information: Tero works with low-resolution images (after all, latency is only a tiny part of a game’s user interface (UI)) that can potentially contain foreign elements. Tero uses open source Optical Character Recognition tools with game-specific UI-based heuristics to avoid wrong measurements.
- Obtaining referential locations: Latency from gaming footage does not have any information about the location from which it was measured. To get this information, Tero uses the descriptions streamers often leave on their Twitch channel description or other social media platforms with a dedicated location field. To extract referential locations from these sources, Tero uses a combination of open-source Natural Language Processing (NLP) tools, comparing their outputs for better accuracy.
In our paper, we provide a more detailed explanation of all the challenges we faced and how we tackled them.
A Look into Latency from Gamers’ Eyes
Using Tero’s data, we can look into Internet latency as experienced by gamers. We look into differences in latency performance in different geographical locations; to keep comparisons fair, we only compare latency at similar distances from their respective servers.
We found surprisingly poor latency performance in certain regions in the United States and Canada compared to users in Brazil. Figure 3 shows the latency distribution obtained for users between 1,000 and 1,500 km to their game server: the latency performance in several US states differs by as much as 30 ms from the rest of the locations – a significant difference for a latency-sensitive application such as gaming.
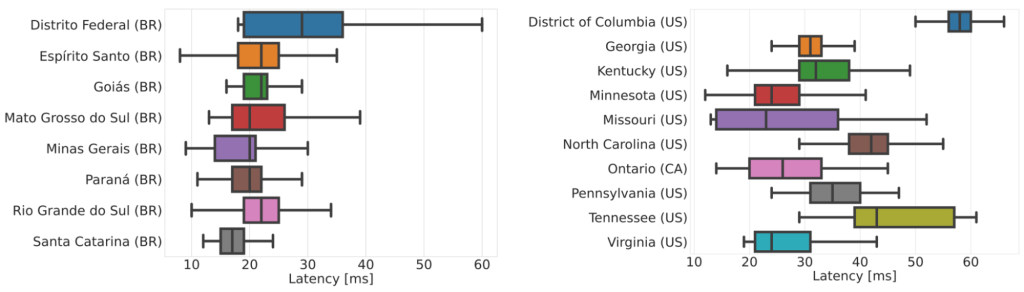
How do Gamers Respond to Latency?
It is common knowledge that video game players care about the latency they experience while playing. However, due to the lack of data, it is hard to predict how they will react to changes in their network conditions. We think that Tero’s data opens the door to research that seeks to answer the question: how do players respond to changes in their latency?
We’ve taken some small steps towards that goal by studying if spikes – increases in the latency experienced by a user – influence players to change the game they are playing. We found a correlation between experiencing more spikes and changing games – the results are similar to other studies on the effects of network conditions on user behavior.
Towards Open Data
The latency obtained by Tero is an opportunity to support the Internet community by leaving it openly available. Therefore, we take extreme care to protect the privacy of streamers by completely anonymizing all data but encourage any streamer with questions about the dataset to reach out to us.
Further, we invite everyone interested in learning more to check out our website or IMC paper.
Catalina Alvarez is a PhD Candidate at EPFL, Lausanne, working in the Network Architecture Lab under the supervision of Prof. Katerina Argyraki. Her research focuses on Internet transparency and leveraging social media to bridge the gap between network metrics and users’ experience.
Photo by Erik Mclean on Unsplash